Understanding Machine Learning: What Is It and Why It Matters for Business Success
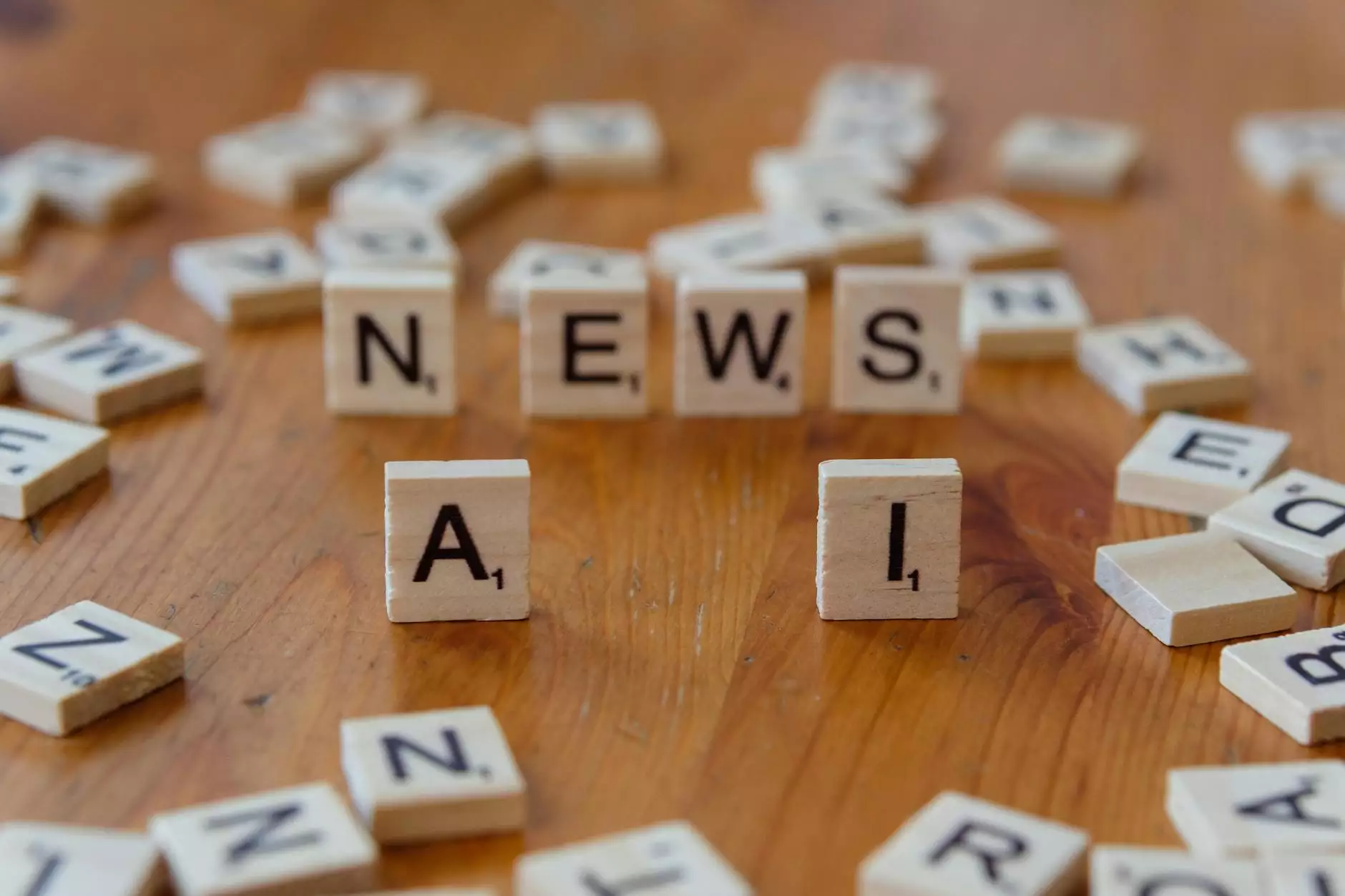
Introduction to Machine Learning: A Fundamental Business Innovation
In today's rapidly evolving digital economy, machine learning has emerged as a transformative force driving innovation across industries. But for many, the question remains: machine learning what is? At its core, machine learning is a subset of artificial intelligence that enables computers to learn from data and make decisions or predictions without being explicitly programmed. This powerful technology is revolutionizing how businesses operate, compete, and innovate.
The Definition of Machine Learning: An In-Depth Explanation
Machine learning can be described as the process by which algorithms automatically improve through experience. Unlike traditional software models that follow explicitly coded instructions, machine learning models identify patterns within data to generate accurate predictions and insights. This results in a dynamic, adaptive system capable of handling complex, unstructured, and voluminous data streams.
Specifically, machine learning involves training models on large datasets, allowing the system to recognize patterns and relationships. Once trained, these models can make predictions, classify information, and even generate new data. The key attributes of machine learning include:
- Data-driven learning: Utilizing vast and varied datasets to improve system performance.
- Adaptability: Continuously updating models with new data for improved accuracy.
- Automation: Eliminating manual oversight in decision-making processes.
- Predictive analytics: Anticipating future events based on historical data.
Types of Machine Learning: Tailored Solutions for Diverse Business Needs
Understanding the different types of machine learning is vital for deploying it effectively. Broadly classified, these types include:
Supervised Learning
In supervised learning, models are trained using labeled datasets—meaning each data point is annotated with the correct output. This approach excels in tasks like fraud detection, customer segmentation, and demand forecasting, where historical data informs future predictions.
Unsupervised Learning
Unsupervised learning involves examining unlabeled data to find hidden patterns or groupings. Clustering algorithms are common here, used in customer segmentation, anomaly detection, and recommendation systems.
Semi-supervised and Reinforcement Learning
Semi-supervised approaches blend labeled and unlabeled data for situations where labeling is costly or impractical. Reinforcement learning, on the other hand, enables systems to learn optimal behaviors through trial and error, akin to training a dog with rewards and penalties. These advanced methods open new horizons for complex decision-making and autonomous systems.
Real-World Business Applications of Machine Learning
Now that we understand machine learning what is, it’s crucial to see how it is applied in real-world business contexts to achieve competitive advantages:
Customer Experience and Personalization
- Recommendation engines: Netflix, Amazon, and Spotify utilize machine learning to personalize content and product suggestions based on user behavior.
- Chatbots and virtual assistants: AI-powered customer service bots deployed by brands like Bank of America and H&M enhance user interaction 24/7, reducing operational costs and improving satisfaction.
Operational Efficiency and Process Automation
- Supply chain optimization: Machine learning models predict demand, optimize inventory, and streamline logistics.
- Predictive maintenance: Manufacturing firms like GE and Siemens use machine learning to forecast equipment failures and schedule timely maintenance, minimizing downtime.
Fraud Detection and Risk Management
- Financial services: Banks leverage machine learning algorithms to detect fraudulent transactions in real-time and assess credit risk more accurately.
- Insurance: ML models analyze claims data to detect anomalies and prevent fraud, saving millions annually.
Healthcare and Biomedical Innovation
- Diagnostics: Machine learning models analyze medical images and genomic data for early detection of diseases like cancer.
- Drug discovery: Accelerating research by predicting molecular interactions and clinical trial outcomes.
The Business Advantages of Embracing Machine Learning
Implementing machine learning solutions offers a plethora of benefits that empower organizations to innovate, improve, and stay ahead:
- Enhanced Decision-Making: Data-driven insights lead to more accurate and timely decisions.
- Increased Revenue: Personalized marketing and improved operational efficiency boost sales and profitability.
- Cost Reduction: Automating routine processes reduces labor and operational costs.
- Competitive Differentiation: Early adoption of machine learning fosters innovation and a unique market position.
- Improved Customer Loyalty: Personalized experiences strengthen customer relationships and retention.
How to Get Started with Machine Learning in Business
For organizations eager to explore machine learning what is, building a foundational strategy is essential:
- Identify Business Challenges: Pinpoint areas where data-driven insights can generate significant value.
- Data Collection and Preparation: Gather high-quality, relevant data while ensuring privacy and compliance.
- Invest in Talent and Technology: Hire data scientists, machine learning engineers, or partner with specialized consulting firms like machinelearningconsulting.net.
- Develop and Train Models: Use appropriate algorithms to train solutions tailored to your specific needs.
- Test and Deploy: Rigorously validate models and implement them into real-world operations, continuously monitoring for improvements.
Key Factors for Successful Machine Learning Adoption
While the potential of machine learning is immense, success hinges on several critical factors:
- Data Quality: Robust, clean, and diverse datasets are the backbone of effective models.
- Skilled Talent: Expertise in data science, statistics, and domain knowledge is vital.
- Clear Business Goals: Align machine learning initiatives with strategic objectives for maximum impact.
- Continuous Learning and Optimization: AI models require ongoing updates and improvements as new data becomes available.
- Ethical Considerations: Address privacy, bias, and accountability to build trustworthy AI systems.
Future Outlook: The Evolving Landscape of Machine Learning in Business
As technology advances, machine learning is poised to become even more integral to business operations. Trends such as explainable AI, edge computing, and federated learning are expanding the horizons of what organizations can achieve. Businesses investing in these innovations will enjoy greater agility, deeper insights, and more personalized customer experiences.
Furthermore, the fusion of machine learning with emerging technologies like the Internet of Things (IoT) and blockchain promises to unlock new revenue streams and operational efficiencies. Forward-thinking companies will view machine learning not just as a tool but as a core component of their digital transformation roadmap.
Why Partnering with Experts Matters
Successfully integrating machine learning into your business is no small feat. It requires strategic vision, technical expertise, and a nuanced understanding of your industry. Partnering with experienced consulting providers like machinelearningconsulting.net can accelerate your journey, provide custom-tailored solutions, and ensure ethical and sustainable deployment.
Conclusion: Embracing the Future of Business with Machine Learning
To sum up, machine learning what is is fundamentally about empowering businesses to make smarter, faster, and more informed decisions by harnessing the power of data. Its applications have proven transformative across sectors, enhancing customer experiences, streamlining operations, and unlocking new growth avenues. Businesses that proactively adopt and adapt to machine learning will not only thrive today but will shape the innovations of tomorrow.
Investing in this technology and understanding its potential is more than a strategic advantage—it's a necessity in the competitive landscape of modern enterprise. The future belongs to those who recognize the significance of machine learning and leverage it effectively.